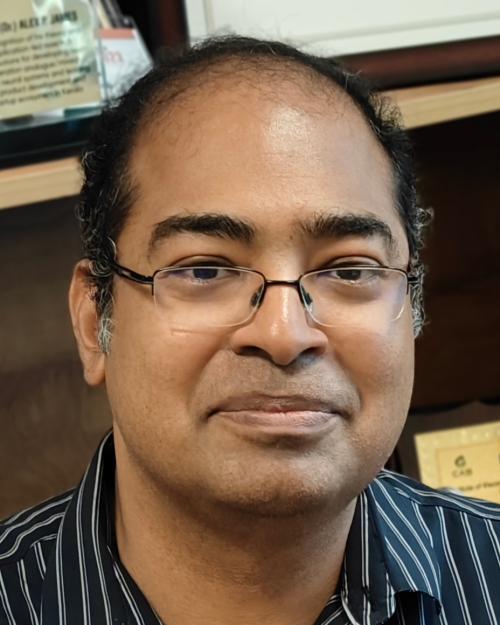
Crossbar based Mixed-Signal Neural Architectures under Variability and Parasitics
Presentation Menu
In this lecture, the variability impact, compensation techniques, and variability-aware neural architectures are discussed. Variability and paracitics pose significant challenges in ensuring accurate multiply and accumulate (MAC) computations emulated with a crossbar. In most neural networks, the MAC forms the core computing module to implement a neuron and, consequently, neural networks. The analog MAC blocks are particularly susceptible to variability and parasitics, which can result in inaccuracies in the computation process. Therefore, developing techniques to mitigate these challenges is crucial for the successful implementation of variability averse mixed-signal neural architectures.