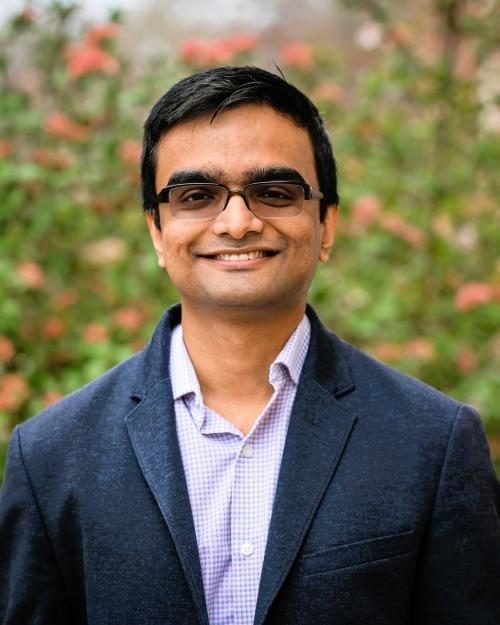
Ferroic Material Based Neuromorphic Computing: A Hardware-Software Co-Design Perspective
Presentation Menu
Deep learning has made significant progress over the past few years in a plethora of cognitive applications. However, despite such advancements, an often underestimated question is the amount of computational resources and energy consumption that these platforms typically require for running on hardware. Bridging the computational efficiency gap necessitates the exploration of devices, circuits, and architectures that provide a better match to the computational primitives of biological processing—neurons and synapses—and which require a significant rethinking of traditional von-Neumann based computing.
Recent experiments in ferromagnetic and ferroelectric devices are revealing immense possibilities of implementing a plethora of neural and synaptic functionalities by single device structures that can be operated with very low energy consumption. Leveraging insights from such experiments, I will present a multi-disciplinary perspective across the entire stack of devices, circuits, and systems to envision the design of a neuromorphic processor enabled with on-chip learning functionalities that can potentially achieve two to three orders of magnitude energy improvement in comparison to state-of-the-art CMOS implementations.
Probabilistic computing paradigms that embrace device-circuit non-idealities instead of viewing them as a disadvantage will also be discussed. I will conclude the presentation by providing my vision of enabling end-to-end cognitive intelligence across the computing stack that combines knowledge from devices and circuits to machine learning and computational neuroscience.