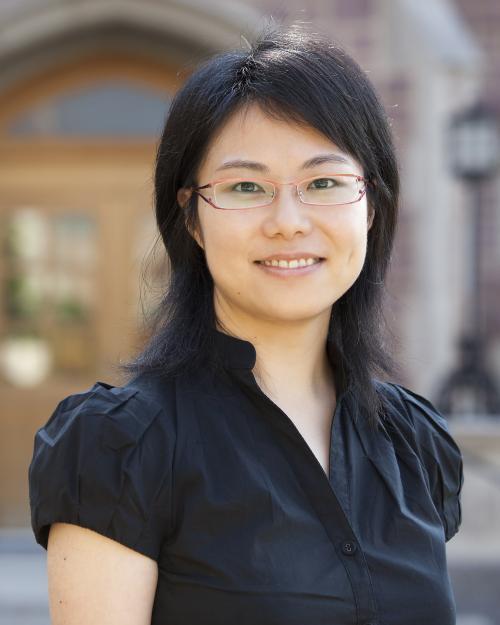
Exploring Autonomous Edge Intelligence in the Analog Domain
Presentation Menu
Recent rapid advancements of artificial intelligence (AI) and machine learning (ML) technologies have fueled many burgeoning smart applications from personalized recommendations to self-driving cars. Despite these unprecedented developments, the holy grail of enabling fully autonomous machine intelligence remains far from our grasp. One key challenge is the lack of performant and efficient hardware implementation to support these computationally demanding tasks, especially on edge devices with stringent resource constraints. In this talk, I will present research from my lab to tackle the edge intelligence challenge from a unique angle that explores system/design abstractions beyond the conventional binary digital logic. Instead, we leverage the signal representation and information processing ability that are innate in the analog/mixed-signal (AMS) domain. Our AMS-domain method not only leads to novel resistive RAM (RRAM) based analog-to-digital converter designs that exceed the state-of-the-art performance limit, but it also transforms the peripheral circuits in in-memory computing (IMC) architectures which are crucial to delivering much-improved computing performance and efficiency. In the end, I will also reveal how an AMS approach can enrich our technological arsenals from several diverse domains such as learning-assisted design automation, cross-domain system security, and real-time and adversarial autonomy.