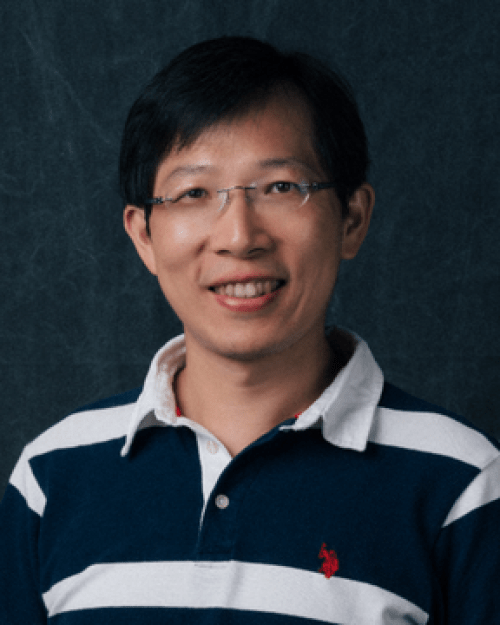
Video/Image Coding for Machines
Presentation Menu
The traditional video/image coding is optimized primarily for preserving the waveforms of video/image signals in a form suitable for human perception. However, the emergence of artificial intelligence (AI)-enabled visual recognition systems often requires video/images (or more broadly, visual data) to be analyzed by machines for image processing and/or computer vision tasks. In many real-world applications, the video/image acquisition happens on resource-limited edge devices, with the compressed bitstream stored or transmitted to the cloud for analytics. This calls for a compact representation of video/images that is optimized not only for human perception but also for machine consumption. The demand has recently kicked off the development of a new type of coding technology in both academia and standards organizations (e.g. JPEG AI and MPEG). In this endeavor, deep learning is emerging as the enabling technology due to its great success in computer vision tasks and learned-based video/image compression. The wide variety of application scenarios makes this rapidly growing field a wide open space for research, inviting contributions from communities of various disciplines. In this talk, I shall first (1) overview the recent advances in this area, particularly the standardization activities taking placing in JPEG and MPEG. In the second part, I shall (2) review some notable designs that adopt end-to-end learned systems (which replace the compression backbone with neural networks) as solutions. The third part (3) explores the learning-assisted approach (i.e. re-purposing or enhancing the traditional codecs by learning techniques without changing the codecs) to coding for machines. Lastly, I shall (4) discuss their circuits and systems implementations for resource-limited applications.